top of page
Every modelling calibration effort, doesn't matter if manually made or if implemented through any kind of automatized routine, is a Bayesian exercise. In other words, the expectations enveloped in any or ever one of its estimatives (the weights [wi], if you please) has, necessarily, the predicative to influence these estimatives.
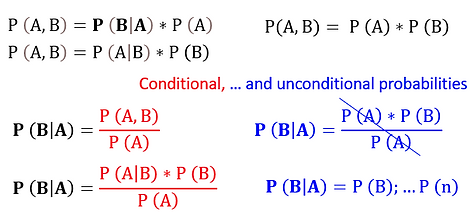
Is meant by Objective Function (Φ), a reference number encompassing what need to be targeted by PEST:

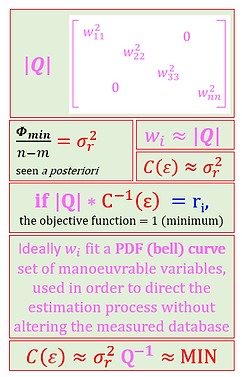

Beta μ
[ ri ] expresses the difference between measured [*Heads] and its calculated counterparts (Xp)
Any residuals of a realization could become neutralized if we knew, beforehand, the noise (ε), the problems, of a database.
So these variables are inversely proportional. Together, they sum-up the current discrepancies (Φ) of a given scenario.
Replacing what is inherently impossible to know ( C(ε) or σr ) before the calibration, to a transitory guess of what that noise might be (as a new kind of variables, the weights [wi]), gives the Objective Function the capability of directing the estimation processes towards what the modeller understands as the most trustful of his information's in a dataset.
In ideal conditions, the exact distribution of disruptive weights in this diagonal matrix (Q) can neutralize all the database noise. In practise, attaching uneven distributions of confidence intervals IN(m3/d, for instance) and margins of error Z(%) to troublesome observations, brings a more feasible task to be overcome by the automatized calibration process (the minimization of original Φ).
bottom of page