top of page
[ h = Z k + ε ] - Each and every model is essentially a crude simplification of reality.
[ h = X p + ε ] - From theoretical idealizations, out there are many more parameters then observations [vector (p) >> vector (h)], "by far".
Manual regularizations (spatial allocation and dimensioning of parameters) narrows the modelling potentialities in the same measure of the assumed simplifications, in a process that is tapered even more, indeed, by the obligatory search of a invertible square X matrix.

A imperative, more realistic approach seeks to embrace not only some well know inherent numerical irregularities, as the heterogeneities of the K variable (this exponential enlargement of numerical field comes coupled with pilot point interpolation strategies).
Also a unprecedent rationalization gain occurs by the accountability of a new kind of "structural noise (η)", beyond the observational data-related ones (ε).
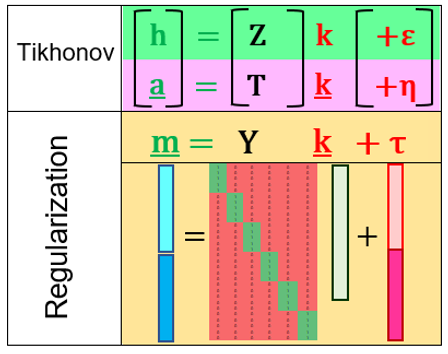
SVD adds handy insights. But SVD-assist holds the prize in easing the estimation process numerical burthen by the identification and use of only the most influential variables as combinations of super-parameters, extending the room for representation of complex scenarios.

Beta μ
bottom of page