top of page
Reinserting (h) in the preceding formulation, we reach a new two-fold error vector (^k).
From now on the already presented calibration noise (ε), gains a not new (or at best not yet unanticipated) error component.
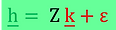
So this new estimation process can be seen carring this kind of "structural" error, ... and the earlier noise, that we still don't know, because we don't know the real parameters. This is the cost of uniqueness of the scalar solution.
Finally, even not knowing the real parameters, comes the innate variability and spatial correlation between these parameters, ... and the noise in the dataset, by a covariance matrix of parameter error (as seen in propagation of variance rule page 13).

Beta μ
P.S. For sake of simplification, as stated in page 13, this example also assume that the noise C(ε) associated with each one and between the measurements are statistically independent and that it have a even standard deviation of σ2k
P.S. Similarly, the covariance matrix C(k) says that the parameters are statistically independent, and that it posses a even standard deviation of σ2ε

bottom of page