top of page
In one hand, it saves SVD of its inability to represent [expert] knowledge constrains in the simple form of a unique coveriance matrix C(k)= σ2kI.
A more
reliable weighting strategy
...
SVD and Tikhonov

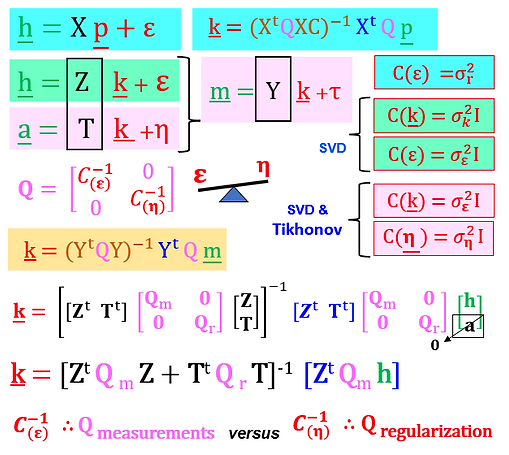
bottom of page